Regional accounts - an analysis of the economy for NUTS level 3 regions
Data extracted in September 2020.
No planned article update.
Highlights
20 % of the EU’s GDP was generated in 86 NUTS level 3 regions, which collectively covered 1.2 % of the EU surface.
Compared with the average for the EU, regional labour productivity increased markedly between 2010 and 2017 in Dublin, South-East (both Ireland), Wolfsburg, Kreisfreie Stadt, Ingolstadt, Kreisefreie Stadt (both Germany) and Pieriga (Latvia).

Source: Eurostat (nama_10r_3gdp) and (nama_10r_3popgdp)
This article provides an analysis of regional accounts data available for the European Union’s (EU) NUTS level 3 regions. Regional data at level 3 provide more granular (detailed) information than the data at level 2, which are the ones most often presented in Eurostat publications. Based on the 2016 version of NUTS, there are 1 169 level 3 regions in the EU-27 compared with 240 level 2 regions.
The use of level 3 data makes it possible to have a regional analysis for several of the Member States that only have a single level 2 region: there are only two Member States — Cyprus and Luxembourg — that also remain a single region at level 3. Furthermore, level 3 offers a more enlightening regional analysis for Member States with only two or three regions at level 2, namely Ireland, Croatia, Lithuania and Slovenia. For these Member States, a level 2 analysis may well be particularly unbalanced due to the dominance of the region that contains the capital city; note that this can also happen at level 3 for Member States with only two or three regions. Equally, the information available at level 3 is much more detailed than that at level 2 for some of the larger Member States. Most notably, Germany has 401 level 3 regions compared with just 38 level 2 regions.
While a regional analysis is more detailed at level 3 than at level 2, data are available for far fewer national accounts variables. For example, there are no regional accounts data on the compensation of employees, hours worked, investment or household accounts. The information available from regional accounts for level 3 concerns gross domestic product (GDP) and population, as well as a relatively aggregated activity analysis (depending on the Member State, either for 6 or for 10 activities according to NACE) for gross value added and employment.
Statistics on regions — the NUTS classification
At the heart of regional statistics is the NUTS classification — a classification of territorial units for statistics. This regional classification for EU Member States is based on a hierarchy of regions and subdivides each Member State into regions that are classified according to three different levels, covering levels 1, 2 and 3 from larger to smaller areas. Some EU Member States have a relatively small population and may therefore not be subdivided at some (or even all) of the different levels of the NUTS classification. For example, Estonia, Cyprus, Latvia, Luxembourg and Malta are each composed of a single level 2 region according to the 2016 version of the classification. For non-member countries covered in this article — the United Kingdom, EFTA countries and some candidate countries — the concept of ‘statistical regions’ is used instead of NUTS. This applies principles analogous to those used in the establishment of NUTS, but is based on gentlemen’s agreements between the countries concerned and Eurostat (rather than having any legislative basis). Table 1 provides an overview of the number of NUTS and statistical regions in each Member States as well as the above mentioned non-member countries.
Full article
Regional concentration of economic activity within the EU
Map 1 shows the regional concentration of economic activity within the EU. For each region, the GDP per inhabitant (also referred to as GDP per capita) has been calculated based on GDP data presented in purchasing power standards (PPS).
GDP in PPS
GDP expressed in PPS is calculated by applying purchasing power parities, the latter being indicators of price level differences between countries. The use of purchasing power parities rather than market exchange rates to convert currencies makes it possible to compare the output of economies and the material welfare of their inhabitants in real terms, in other words controlling for differences in price levels. By convention, for the EU as a whole, GDP in PPS has the same value as GDP in euro; these values (in PPS and euro) are different for individual EU Member States and regions, depending on the underlying price level differences.
GDP per inhabitant
Presenting the data as a ratio to the number of inhabitants in each region standardises the data to take account of the different size (in population terms) of each region.
The 1 167 regions for which data are available have been sorted based on the value of their GDP per inhabitant. They have then been grouped into five roughly equal parts, each accounting for approximately PPS 2.6 trillion (thousand billion) of GDP in 2017, in other words one fifth (20 %) of the EU-27’s total GDP. Just 86 level 3 regions made up the first fifth of the EU economy in 2017. These include 63 German regions (which together contributed 38 % of the total GDP for this first group), five Austrian regions, four Dutch regions, two regions each from Denmark, France and Italy, and one region each from Belgium, Czechia, Ireland, Luxembourg, Poland, Romania, Slovakia and Sweden. These 86 regions cover an area of approximately 52 000 square kilometres, representing 1.2 % of the total area of the EU. The other groups (second to fifth) are composed of 133, 208, 317 and 423 level 3 regions respectively.

Source: Eurostat (nama_10r_3gdp) and (nama_10r_3popgdp)
There are two issues to keep in mind when using GDP per inhabitant measured in PPS as an indicator of the economic situation in a region. First, for certain EU Member States and/or regions, commuting flows are very important. The economic activity taking place in region A may result from the work of many workers living in a neighbouring or nearby region B, which may even be in another Member State. Dividing the GDP of region A by the population of region A, results in a GDP per inhabitant of region A which appears to be relatively high. Two neighbouring level 3 regions in Germany — Wolfsburg and Helmstedt — illustrate this situation. Wolfsburg was the level 3 region with the highest GDP per inhabitant in 2017 in the EU, at 155 000 PPS, while the neighbouring region of Helmstedt had a GDP per inhabitant of 18 000 PPS. This difference in GDP per inhabitant — 8.5 times as high in Wolfsburg as in Helmstedt — is mainly explained by commuting flows.
Secondly, the use of national PPS (as regional ones are not available) overstates the GDP per inhabitant in PPS in relatively expensive regions within any given Member State, while the values for relatively cheaper regions within a Member State are understated. The French regions of Paris and Mayotte are good examples. Applying the national PPS underestimates the price level in Paris and overestimates the price level in Mayotte. There are no official estimates of regional variations in price levels across the EU. However, estimates based on detailed data for consumer prices in Germany indicate that the regional variation may be as high as 26 %, between level 3 regions, in particular, due to housing costs [1].
Bearing these issues in mind, Table 2 shows what percentage of the GDP of each EU Member State belonged to each of the five groups (based on the value of GDP per inhabitant in each region) for the year 2017. Looking at the bottom 20 % group, it includes regions that represent around 60 % of the national economy in Bulgaria, Greece, Croatia, Hungary, Poland, Romania and Slovakia and around 20 % of the national economy in Spain, France (2016 data) and Italy. The impact of commuting is clearly visible in the capital regions of Czechia, Slovakia and Poland, which are part of the top 20 % group while the rest of the regions of those countries are included in the two bottom groups.
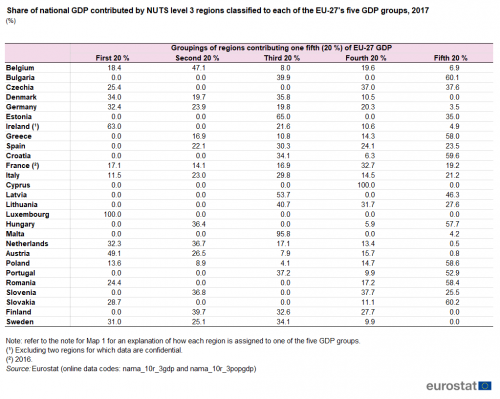
(%)
Source: Eurostat (nama_10r_3gdp) and (nama_10r_3popgdp)
Another interesting aspect of the concentration of regional economic activity is to analyse the developments observed over a number of years. Map 2 classifies the level 3 regions for which data are available according to two different criteria: whether their share of EU-27 GDP decreased or increased between 2010 and 2017 and whether their share of national GDP decreased or increased during the same period. By definition, non-member countries do not have any share of EU-27 GDP, and so the values shown for regions in these countries reflect the change in the ratio of their GDP to the EU-27’s GDP.
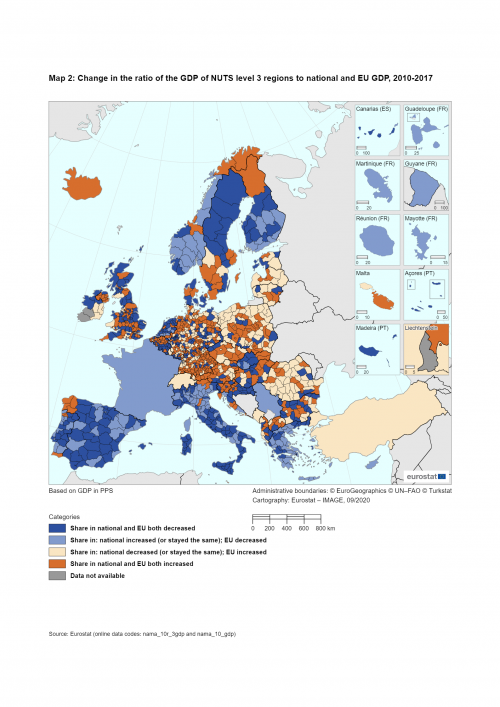
Source: Eurostat (nama_10r_3gdp) and (nama_10r_3popgdp)
None of the Greek level 3 regions increased their share in the EU-27’s GDP between 2010 and 2017, while this share increased only in four regions in Spain, three regions each in Italy and Portugal, two regions in Slovakia and one region in Finland. By contrast, 12 Czech regions, 302 German regions, six Latvian regions, eight Lithuanian regions, 65 Polish regions and 38 Romanian regions increased their share in the EU-27’s GDP during this period.
Looking at the share in national GDP (rather than in the EU-27 total), 20 out of 28 Bulgarian regions saw their share of national GDP decrease, as was the case for four of the five Estonian regions, 42 of the 60 Spanish regions, 8 of the 10 Lithuanian regions, five of the seven Latvian regions and 33 of the 43 Romanian regions. In most cases, the decreases in the shares were explained by an increase in the more developed regions of those Member States.
Regarding the level 3 regions that were in the top 20 % of the EU economy (as shown in Map 1), increases in the share of national GDP were observed for several regions, including many capital city regions and other relatively wealthy urban areas, such as Groot-Amsterdam (the Netherlands), Bucureşti (Romania), Stockholms län (Sweden), Byen København (Denmark), Miasto Warszawa (Poland), München, Kreisfreie Stadt (Germany), Milano (Italy) and Bratislavský kraj (Slovakia). However, there were exceptions; some capital city regions were in the top 20 % of the EU economy but experienced decreases in their share of national GDP, as was the case for example in Hlavní město Praha (Czechia), Arr. de Bruxelles-Capitale/Arr. van Brussel-Hoofdstad (Belgium), Wien (Austria) and Dublin (Ireland).
Regional labour productivity
In the first part of this article, regions were initially categorised by their GDP per inhabitant, which is the result of dividing GDP by the population. GDP per inhabitant can also be considered to be labour productivity (GDP divided by employment) multiplied by the proportion of persons employed within the population (employment divided by population).
This section focuses on labour productivity. For the purpose of this analysis, gross value added is used instead of GDP, so the labour productivity is calculated as gross value added per person employed. This is presented in euros rather than in PPS, as gross value added data are not available in PPS; note that the PPS data for GDP are calculated from the expenditure approach for compiling GDP rather than the output approach which is used as the basis for data on gross value added. Another factor to consider before interpreting the results is that the average hours worked per person varies considerably across EU Member States and economic activities. Persons working in Member States with higher productivity usually work fewer hours than persons employed in Member States that display lower levels of labour productivity. Finally, the productivity of an economy as a whole reflects the productivity of its different economic activities (implicitly weighted by the number of persons employed in each activity). A region specialised in activities with a higher labour productivity will have a higher overall productivity than a region specialised in activities with a lower labour productivity, even if the productivity for each activity is the same in both regions.
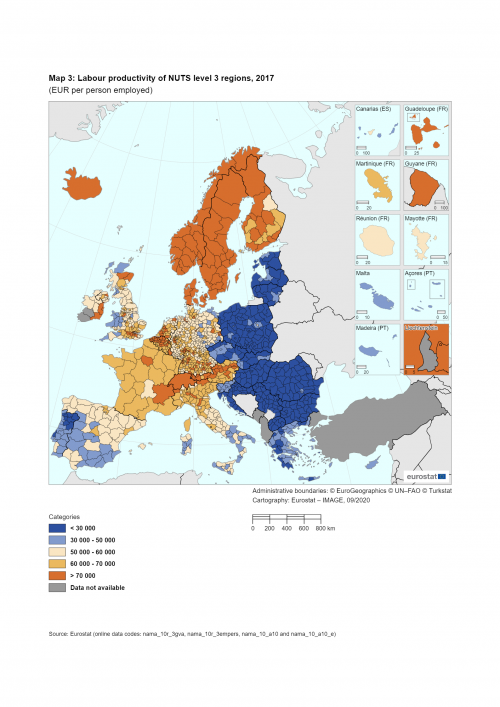
(EUR per person employed)
Source: Eurostat (nama_10r_3gdp) and (nama_10r_3popgdp)
Map 3 shows a distribution of regional gross value added based on a region’s labour productivity. The first step was to calculate the labour productivity for each level 3 region. Five classes of labour productivity were defined, each containing approximately the same number of level 3 regions, starting from the highest of EUR 70 000 or more of gross value added per person employed down to the lowest where labour productivity was less than EUR 30 000. Once each region had been assigned to one of these five classes based on its labour productivity, the value added of the regions within each class were combined; from this value a distribution of national gross value added was calculated. Map 3 clearly shows the differences in labour productivity measured in euros between the regions in the northern and central regions and compared to southern and eastern regions.
Table 3 shows what share of value added was contributed by regions classified to each of the labour productivity classes. The Member States that recorded at least three quarters of their value added in the most productive regions were the Nordic Member States (Denmark, Finland, Sweden), Luxembourg, Belgium, Ireland and Austria, while a majority of value added was also generated in regions with high productivity in the Netherlands. France, Germany and Italy were the only other Member States with any regions that had an average labour productivity of at least EUR 70 000 per person employed. Compared with GDP per inhabitant in PPS in Table 1, the differences in productivity between level 3 regions within a Member State are much smaller.
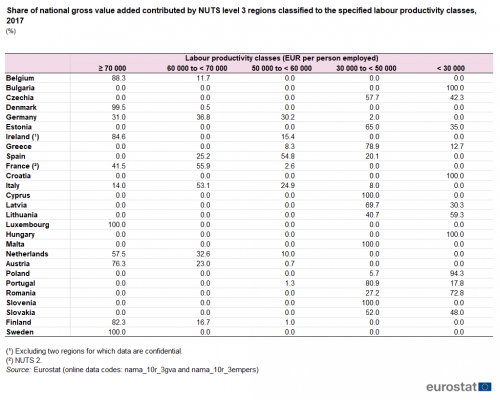
(%)
Source: Eurostat (nama_10r_3gva) and (nama_10r_3empers)
For each level 3 region, Figure 1 shows the change between 2010 and 2017 in a productivity index that has been calculated relative to the EU-27 average. After deriving the level of labour productivity as described above, a ratio was calculated to show the labour productivity of each region relative to the average for the EU-27, with this ratio expressed as a percentage; by definition for the EU-27 the productivity index = 100. The percentage point difference in this ratio for 2010 and 2017 was calculated and this is shown in the figure.
Between 2010 and 2017, all except for one of the level 3 regions in Bulgaria reported an increase in labour productivity compared with the EU-27 average. In most Bulgarian regions, the increase was quite limited and in 21 Bulgarian regions the relative productivity remained below 20 % of the EU-27 average. The relative labour productivity index increased in all regions of Romania, both regions in Malta and the single region of Luxembourg. It also increased in a majority of regions in Belgium, Denmark, Germany, Estonia, Latvia, Lithuania, Austria, Finland and Sweden, as well as in North Macedonia. By contrast, relative labour productivity decreased in every region of Italy and in nearly all of the regions in Greece, Spain and Slovakia.
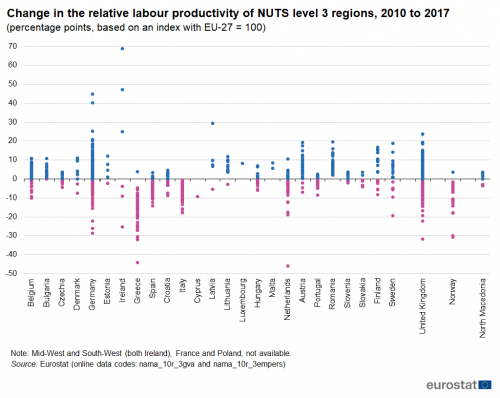
(percentage points, based on an index with EU-27 = 100)
Source: Eurostat (nama_10r_3gva) and (nama_10r_3empers)
The regions with the largest increases in relative labour productivity were Dublin (Ireland; an increase of 69 percentage points), South-East (Ireland; 47 percentage points), Wolfsburg, Kreisfreie Stadt (Germany; 45 percentage points), Ingolstadt, Kreisefreie Stadt (Germany; 40 percentage points) and Pieriga (Latvia; 29 percentage points). With the exception of Pieriga, all were regions that had a labour productivity in 2010 that was already above the EU-27 average. At the other end of the range, the largest decreases in relative labour productivity were recorded in Overig Groningen (the Netherlands; a decrease of 46 percentage points), the Greek regions of Ithaki, Kefallinia (44 percentage points), Andros, Thira, Kea, Milos, Mykonos, Naxos, Paros, Syros, Tinos (32 percentage points), Thesprotia (31 percentage points) and Chalkidiki (30 percentage points), and Wesermarsch (Germany; 29 percentage points). With the exception of Thesprotia, all of these regions also had above average relative labour productivity in 2010. It should be noted that Overig Groningen has a large gas extraction activity. Value added (and therefore labour productivity) in this activity (as well as some of its support activities) is strongly linked to the gas (and indirectly the oil) price which is relatively volatile. In fact, relative labour productivity in Overig Groningen increased by 16 percentage points between 2010 and 2013.
Regional specialisation
The final section of this article looks at regional specialisation of level 3 regions. For this purpose the Krugman specialisation index is used. The index measures the extent to which the structure of a region’s output (based in this case on value added) differs from the average for the EU-27. If the index is close to zero, it implies that the economic structure of the region is very similar to that of the EU-27. The structure has been analysed based on six economic activities which collectively cover the whole economy and for which data are available for all level 3 regions.
There are 214 level 3 regions in the EU for which the Krugman index was above 0.40. These relatively high levels can, at least in part, be explained by a high degree of specialisation in industry, agriculture, finance, tourism-related activities or government. An index above 0.40 was observed for a relatively high proportion of regions in Czechia (9 out of 14), Lithuania (6 out of 10), Hungary (12 out of 21) and Bulgaria (12 out of 28) regions.
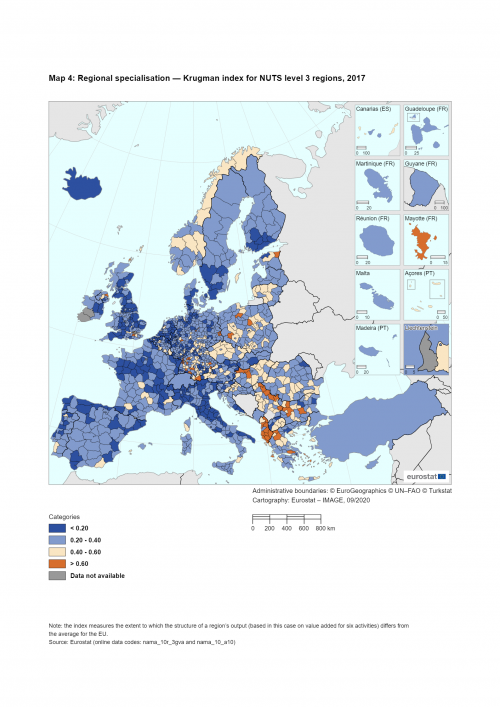
Source: Eurostat (nama_10r_3gva) and (nama_10r_3empers)
Source data for tables and graphs
Data sources
The European system of national and regional accounts (ESA 2010) is the latest internationally compatible accounting framework for a systematic and detailed description of the EU economy. ESA 2010 has been implemented since September 2014 and is consistent with worldwide guidelines on national accounting, as set out in the system of national accounts (2008 SNA).
ESA 2010 ensures that economic statistics for EU Member States are compiled in a consistent, comparable, reliable and up-to-date way. The legal basis for these statistics is a Regulation of the European Parliament and of the Council on the European system of national and regional accounts in the European Union (Regulation (EU) No 549/2013). ESA 2010 is not restricted to annual national accounting, as it also applies to quarterly and shorter or longer period accounts, as well as to regional accounts. It is harmonised with the concepts and classifications used in many other social and economic statistics (for example, statistics on employment, business or international trade) and as such serves as a central reference for socioeconomic statistics.
Context
In her political guidelines for the European Commission for 2019-2024, President van der Leyen highlighted a desire to strive for a European economy that works for people, based on social fairness and prosperity. To do so she called, among other actions, for a deepening of economic and monetary union, greater support for small and medium-sized enterprises (SMEs), the full implementation of the European pillar of social rights, and fairer taxation.
Notes
- ↑ Weinand, S. & L. von Auer (2019): Anatomy of regional price differentials: Evidence from micro price data, Discussion Paper 4/2019, Deutsche Bundesbank, Frankfurt am Main.
Direct access to
- Regional economic accounts - ESA 2010 (t_reg_eco)
- Regional economic accounts - ESA2010 (t_nama_10reg)
- Regional economic accounts (reg_eco10)
- Gross domestic product indicators (reg_eco10gdp)
- Regional economic accounts (nama_10reg)
- Gross domestic product indicators (nama_10r_gdp)
- Branch and household accounts (nama_10r_brch)
- Maps can be explored interactively using Eurostat’s statistical atlas (see user manual)
- Regional statistics illustrated
- Regions in Europe — statistics visualised is a digital publication that offers a range of interactive visualisations that allow you to get a deeper understanding of the situation across European regions
Manuals and further methodological information
- ESA 2010 — manuals and guidelines
- Manual on regional accounts methods — 2013 edition
- Methodological manual on territorial typologies — Eurostat — 2018 edition
Metadata
- Regional economic accounts (ESMS metadata file — reg_eco10_esms)
- European Commission — Economic and financial affairs
- European Commission — EU regional and urban development
- European Commission — European Structural and Investment Funds
- European Commission — InvestEU programme (2021-2027)
- European Commission — New cohesion policy
- European Commission — 2030 agenda for sustainable development